Top 5 AI tools for fast surgical video annotation in 2023
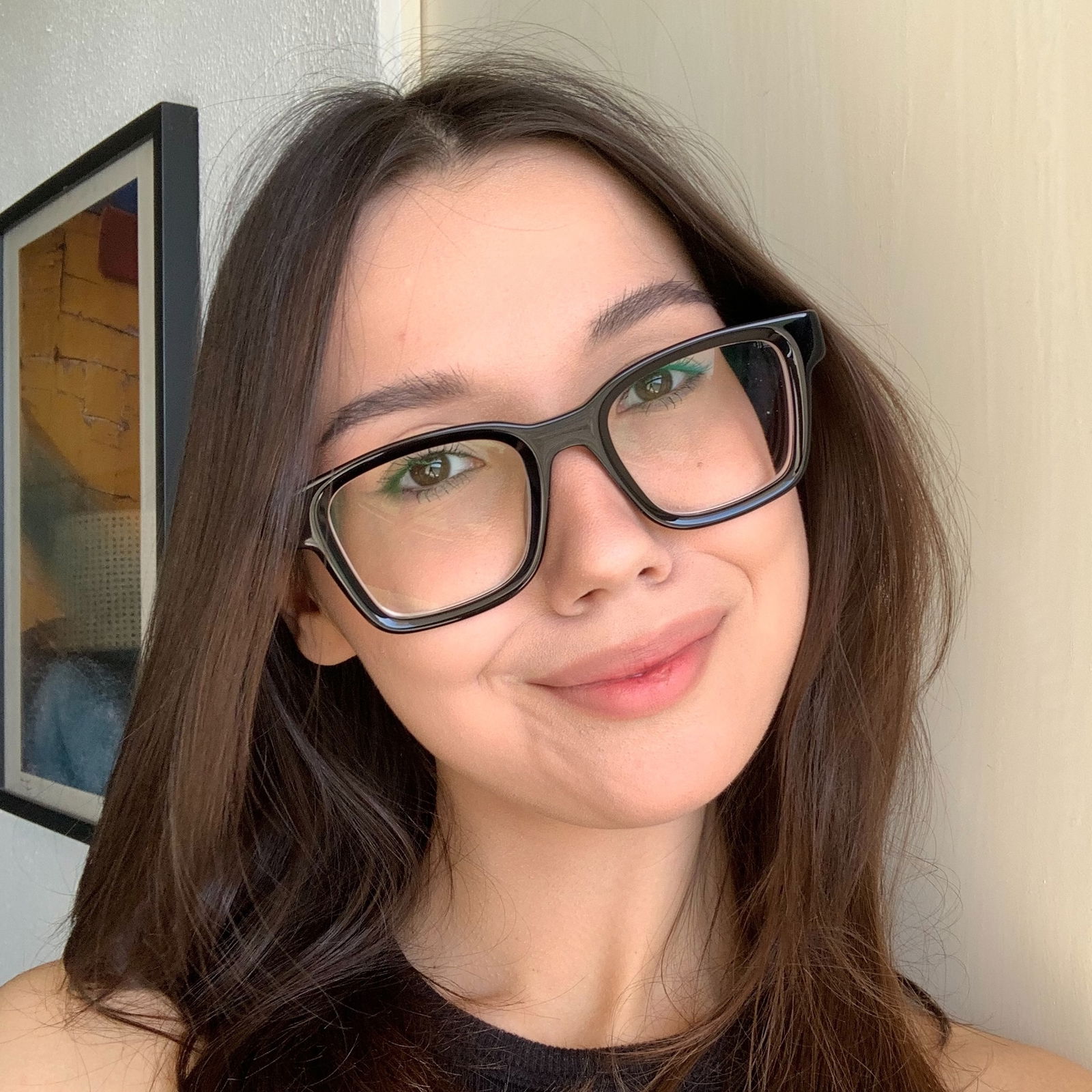
How to use modern AI object tracking and segmentation tools to get medical training data 10x faster
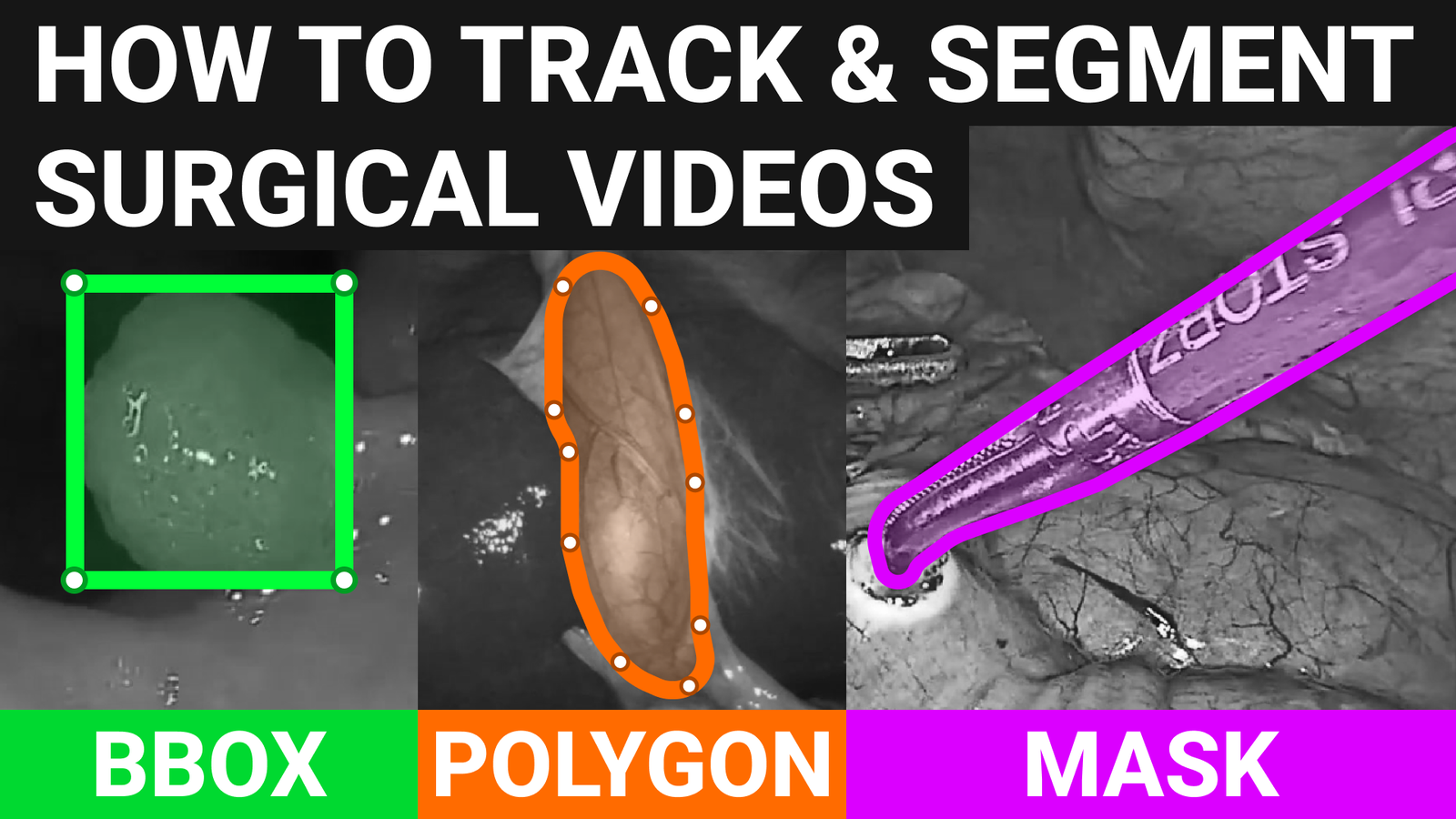
Table of Contents
Why is AI-powered video labeling important?
We understand that the time of domain experts in the medical and healthcare industries is a valuable resource. Surgical video labeling is enormously time-consuming and expensive. So we at Supervisely are developing enterprise-grade computer vision tools that meet high standards in clinical operations and significantly increase annotation productivity by leveraging artificial intelligence. Thus, our customers can achieve data annotation speedups of 10 times or even more and make the training data cost-efficient.
Here is an example of a result you can achieve with our AI-powered segmentation and tracking tools:
Our medical-grade video labeling and clinical operations toolbox will help you achieve success in annotating surgical videos with minimal effort. Our customers are the leading companies in the medical and healthcare industries and we are working closely in collaboration with them to provide powerful computer vision tools for fast training data annotation and neural network training in all modalities, including images, videos and medical DICOM volumes. Thereby Supervisely Platform accumulates best practices and has been designed for surgical data science and surgical intelligence use cases, such as AI-assisted surgery and surgical robotics.
How to speed up manual labeling?
In this tutorial, you will learn how to use Supervisely video labeling tools powered by state-of-the-art (SOTA) neural networks for automatic object tracking, detection and segmentation on videos with bounding boxes, masks and polygons. In addition, we will demonstrate collaboration tools and instruments for assigning tags to video segments for classification and action recognition tasks.
The video guide below from our expert demonstrates the power of model-assisted labeling tools in Supervisely using examples from real-world surgical videos.
1. Bounding box detection and tracking
Modern medicine strives for the continuous development and application of advanced AI technologies that can enhance the accuracy and efficiency of surgical procedures. One such technology that plays a crucial role in modern surgery is model-assisted object detection and tracking.
On the backend, one of the latest SOTA object tracking neural networks MixFormer introduced at CVPR 2022 integrated into Supervisely as well as other models.
MixFormer object tracking
CVPR2022 SOTA video object tracking
One of the key features is that this model is class-agnostic and can track any objects out of the box from different domains without the need for training or finetuning for your specific class.
For example, according to this research colon polyps tracking is a pervasive problem. See how our tool can track such objects:
With the bounding boxes it is easy to highlight the surgical field, instruments and anatomical structures, including tumors, polyps, bleeding areas, resections or human body organs.
Model-assisted video object tracking tools work in the following way. The annotator has to manually put the bounding box around a target object on the selected frame and then run the tracking algorithm to automatically detect an object on the next frames. It is worth noting that at any moment labeler can manually correct an auto-generated bounding box and restart tracking from that moment. Therefore, AI tracking will replace auto-generated objects on subsequent frames with new ones.
Another active research area is the task of surgical tools detection and tracking objects on laparoscopic videos. (image credit, video credit):
Different types of surgical instruments have to be tracked on laparoscopic videos
2. Masks for segmentation and tracking
Masks can be manually labeled with traditional instruments such as brush or pen tools. Supervisely video annotation toolkit supports these basic features. But it is hard and time-consuming to segment objects over thousands of frames. For that purpose, we introduced the SmartTool instrument for model-assisted object segmentation and tracking.
All available SOTA interactive segmentation models are integrated into the Supervisely platform, including:
-
Segment Anything model (SAM) from Meta AI
-
RITM from Samsung AI
-
ClickSeg from Alibaba
-
EiSeg from PaddlePaddle.
-
We constantly add new models that come up in the Machine Learning community.
Our customers can use all of them on the backend of SmartTool.
SOTA interactive segmentation models in Supervisely
Lets consider the example of segmenting instruments used during an operation. SmartTool significantly simplifies the annotation process and reduces the need for manual labeling of each frame individually. Users can interact with the model in real-time and provide feedback with š¢ positive and š“ negative points to correct model predictions.
See in action how to use the interactive segmentation tool to segment and track objects on videos:
SmartTool is a class-agnostic interactive segmentation tool that can segment any object out of the box from different domains. But sometimes it is needed to train or fine-tune these models to adapt them to specific objects to achieve accurate pixel-perfect object masks with as few clicks as possible to save the time of domain experts during the annotation.
There is an app in Supervisely Ecosystem to train a model on custom data. This customization process will be covered in another tutorial on our blog.

Train RITM
Dashboard to configure, start and monitor training
This problem is an active research area and there are several publicly available cataract datasets and many papers.
Segmentation example from cataract datasets
These studies once again prove the importance of automatic object segmentation and tracking in surgery. Video AI labeling toolbox provided by Supervisely platform can significantly help surgical researchers and data scientists to label surgical videos fast and build training data at scale for their machine learning models to push innovations to the next levels.
3. Polygons for segmentation and tracking
Polygons can be used to label objects with complex contours or specific areas of the surgical field. Sometimes it is more convenient to use polygons. For instance, they can assist in highlighting intricate blood vessels, nerve bundles, or regions of interest on organs that can not be adequately represented using bounding boxes.
Supervisely supports polygonal video annotation tools for both manual and automatic labeling and tracking. In 2023 SOTA point tracking model TAP-Net from the Google Deepmind team is integrated into Supervisely and is used as a backend model for tracking polygons on videos.
TAP-Net object tracking
Track points and polygons on videos
Once a polygon is manually created on a selected video frame, interpolation or tracking algorithms can be applied to auto-generate annotations for objects on the next frames. Tracking algorithms are utilized to automatically update the position of each point in the polygon on every frame. This allows users to track object movement on the video segment and create a sequence of annotations with seamless transitions. Additionally, if the polygon tracking model produces errors or inaccuracies, the user has the option to manually adjust and correct the frame and restart the tracking procedure from that moment on. Tracking apps will replace the auto-generated polygons on subsequent frames.
Semantic segmentation made with polygonal annotations of organs and tissues on laparoscopic images and videos is a popular task in surgical AI. Here is an illustrative example of classes that medical researchers label: fat (orange), liver (red), gallbladder (green), instrument (purple), and other (blue).
Laparoscopic image overlayed with its semantic segmentation annotations
4. Action Recognition
Tools for assigning tags and attributes to video segments or entire videos are essential in building training datasets for classification and action recognition models. Such neural networks can be used for the identification of specific events or stages of the operation, like tissue resection moments, specific manipulations with surgical tools like scissors, cutting a polyp, or other significant occurrences. The main objective is to identify and classify these actions, enabling a better understanding of the surgical intervention techniques.
In the example below the tag is assigned to the video segment to mark the existence of a steam on the frames during the full (Roux-En-Y) gastric bypass operation.
5. Collaboration tools
Supervisely platform also provides advanced features for smooth user collaboration, data management, quality control and assurance. It allows defining user permissions to organize the work of members with different roles and expertise - from annotators, reviewers and their managers, to domain experts and data scientists.
Some of the collaboration features in Supervisely platform
By creating labeling jobs and managing access to annotated data and other assets, companies can focus on organizing workflows within a single environment instead of maintaining and integrating multiple in-house or commercial tools.
You can learn more about how to perform collaboration on the Supervisely platform in our full computer vision course.
Data privacy and enterprise-grade
Supervisely platform offers a complete solution to our customers with all necessary enterprise-grade features, support and additional complementary services. It covers all steps from data annotation to neural network training and is designed to build computer vision solutions quickly. Learn more about how we work with customers in this video.
Here are the key moments:
-
Supervisely is an on-premise solution that guarantees š data privacy. Customers can install and manage it on their own private infrastructure even in offline mode without internet access.
-
Works directly with the original video files. Supervisely is optimized to stream, render and label video files in all popular formats and does not split video frames into images like other solutions. You can directly connect your data storage to Supervisely without data duplication.
-
Meets the regulatory requirements, HIPAA compliant and supports all needed privacy and security policies, including data encryption, anonymization, activity monitoring, access alerts, vulnerability scanning and many more.
-
Supports both bare metal installation and all cloud providers (AWS, GCP, Azure, ...) and flexible configurations for complex environments, including Kubernetes.
-
Our engineers, developers and data scientists provide priority support and training sessions to make sure our customers keep successfully solving their tasks.
-
Everything is customizable on all levels from import and export to custom labeling interfaces and data pipelines. Visit our developer portal and build your private applications from scratch or by forking and modifying any app from our growing ecosystem of apps.
-
We regularly integrate all modern neural networks and other computer vision tools, so our customers are up to date with the latest AI and can focus on innovations instead of spending time on integration and debugging.
-
Easy maintenance, upgrades and backups.
Conclusion
In surgical operations, precise object tracking and segmentation on videos can become an integral part of robotic surgery development, enabling the transmission of information to a surgical robot for automatic or semi-automatic navigation and high-precision operation execution. By utilizing both basic annotation tools such as masks, bounding boxes and polygons, as well as advanced techniques like AI-assisted smart tools and tracking, surgical videos can be annotated with maximum productivity using Supervisely Platform.
This perspective not only contributes to improving surgery by eliminating factors such as shaky hands or other human-related issues but also facilitates accurate identification of different affected areas during the surgical procedure itself. Such technologies ultimately benefit both surgeons and patients. And we at Supervisely are going to continue our contribution to the Machine Learning community and the surgical AI industry in particular.
Supervisely for Computer Vision
Supervisely is online and on-premise platform that helps researchers and companies to build computer vision solutions. We cover the entire development pipeline: from data labeling of images, videos and 3D to model training.
The big difference from other products is that Supervisely is built like an OS with countless Supervisely Apps ā interactive web-tools running in your browser, yet powered by Python. This allows to integrate all those awesome open-source machine learning tools and neural networks, enhance them with user interface and let everyone run them with a single click.
You can order a demo or try it yourself for free on our Community Edition ā no credit card needed!